Biomarkers in psychiatry remain elusive, and typical BOLD fMRI measures show low reliability. In contrast, moment-to-moment variability in brain activity can reliably index the effectiveness of neural systems, but has so far not been linked to psychiatric treatment response.
In a sample of social anxiety patients, we recorded BOLD during resting state and emotional face viewing (a social anxiety-relevant task) in two fMRI sessions, 11 weeks apart. Afterwards, patients received a 9-week CBT and clinical symptoms were monitored pre and post CBT.
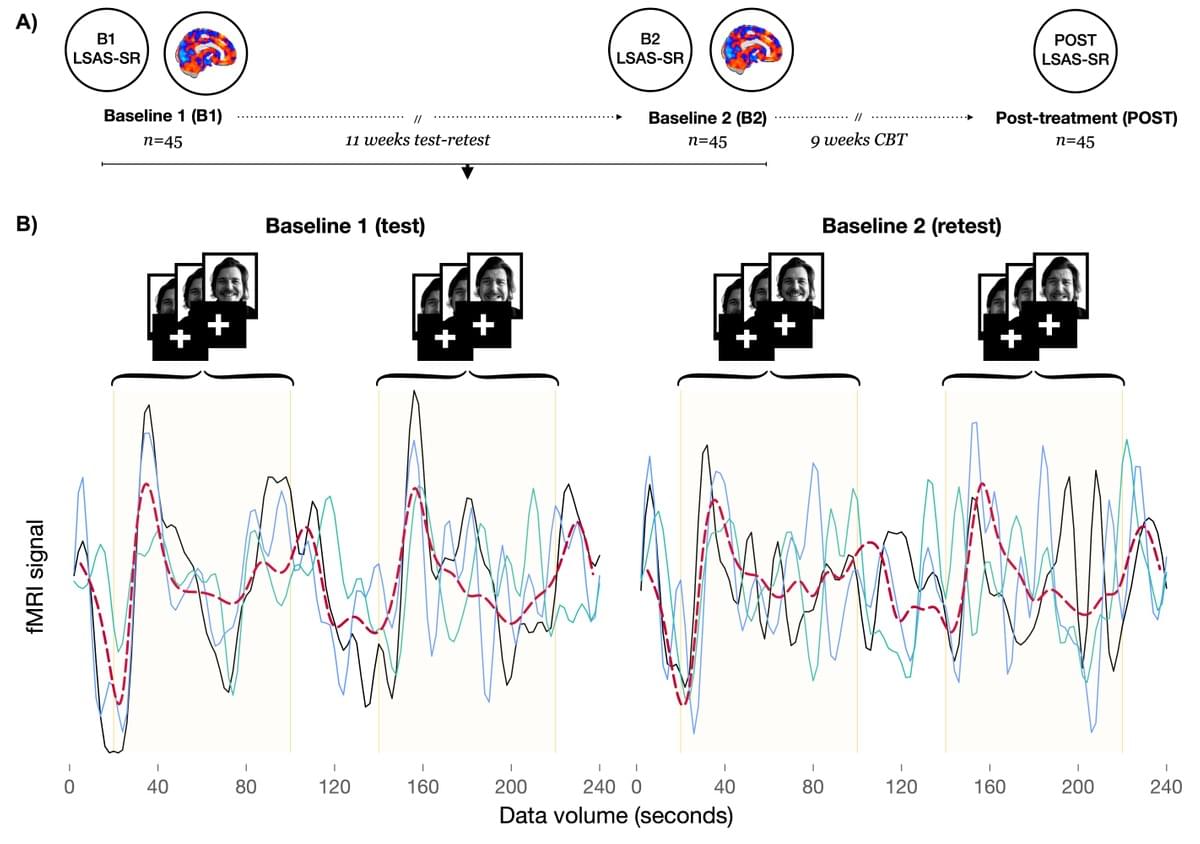
We showed that BOLD variability strongly predicted CBT outcome (r[Predict,Actual] = .77). In particular, task-related BOLD variability was a better predictor than 1) social anxiety self-reports, 2) resting-state variability, and 3) standard mean-based BOLD measures.
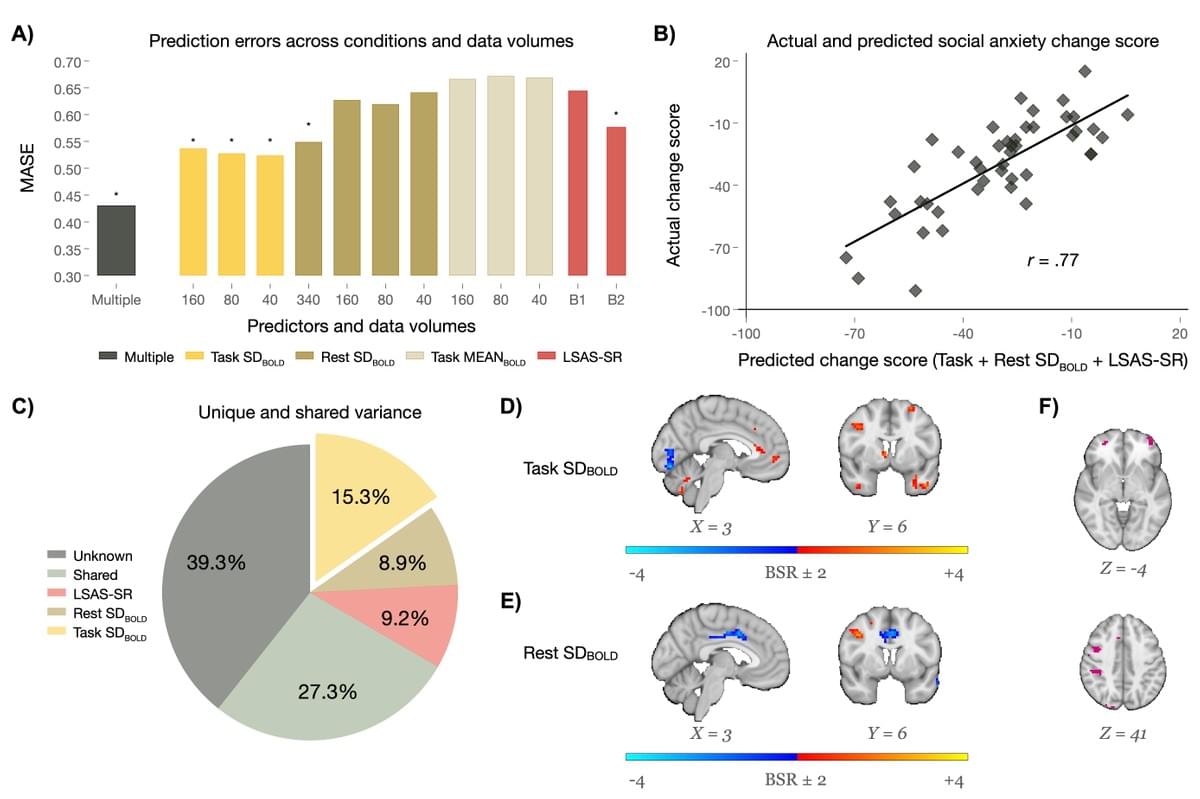
Task-related BOLD variability exhibited excellent test-retest reliability of treatment outcome prediction (ICC=.80). Strikingly, high reliability and prediction accuracy were achieved despite the extraordinarily short face task (<3 min).
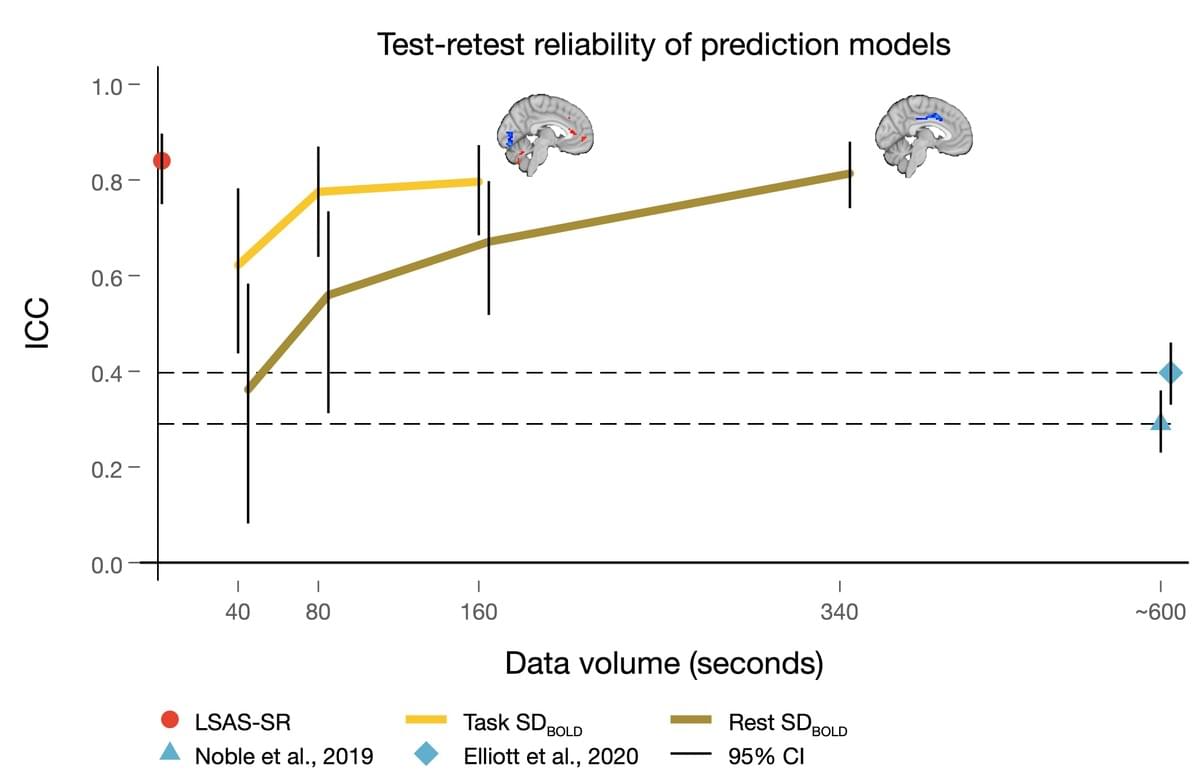
Notably, task-based lower variability in visual cortex was the strongest CBT outcome predictor. What could this mean? These patients may show a heightened focus on emotional content at the cost of an incomplete sensory representation processing.
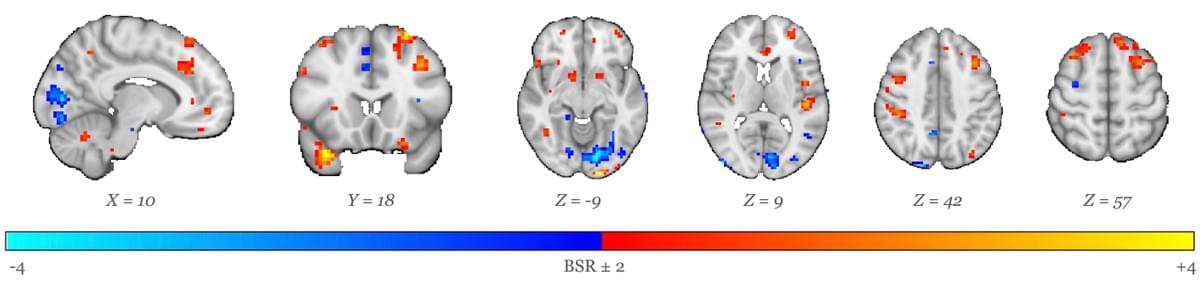
Indeed, lower variability in visual cortex is expected when individuals do not fully process the complexity of the input (Garrett et al., 2020). It is thus plausible that this deficit is targeted by CBT.
BOLD variability shows potential as a viable and efficient treatment outcome predictor, particularly with disorder-relevant tasks. We need to rethink our standard approaches in psychiatric fMRI. Resting-state is simple, but it might miss the boat experimentally.
Our task required passive viewing of faces for minimal time, and yet, it outperformed resting-state in all models (sometimes dramatically). We simply need to have broader discussions about how to design efficient and disorder-relevant treatment prediction studies.
Code and output are available at GitHub. We are also working hard to remove constraints on our ability to openly share patient data. In the meantime, please contact us for alternative routes to data access if interested.
Below you find recorded presentation of our paper: